Dr. Nicola Falco
Research Scientist
Lawrence Berkeley National Laboratory
Earth & Environmental Sciences Area (EESA)
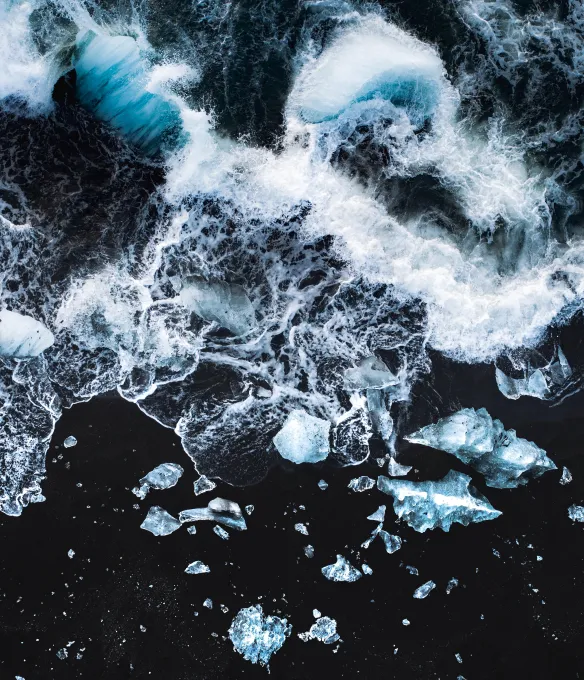





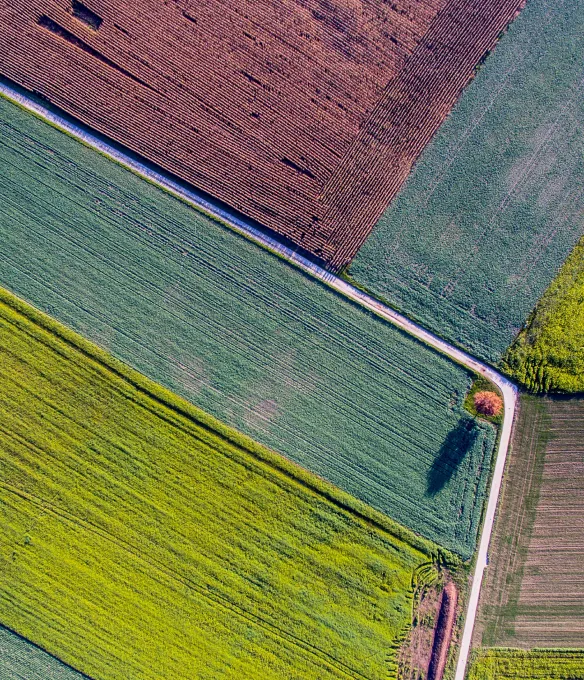
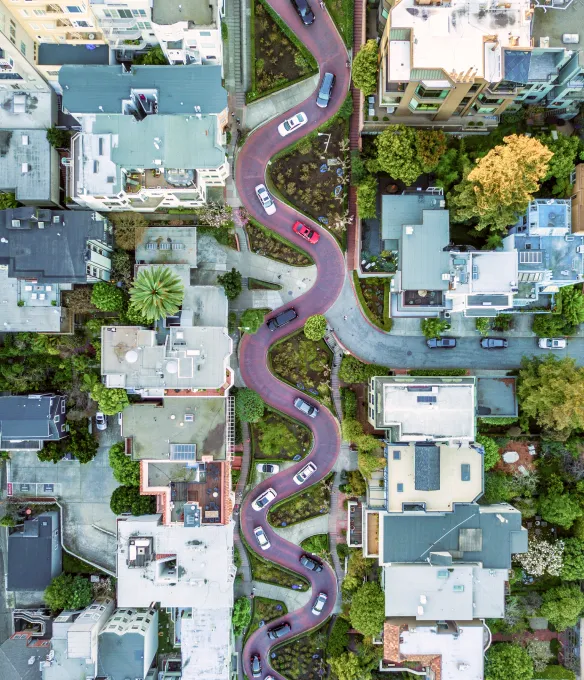

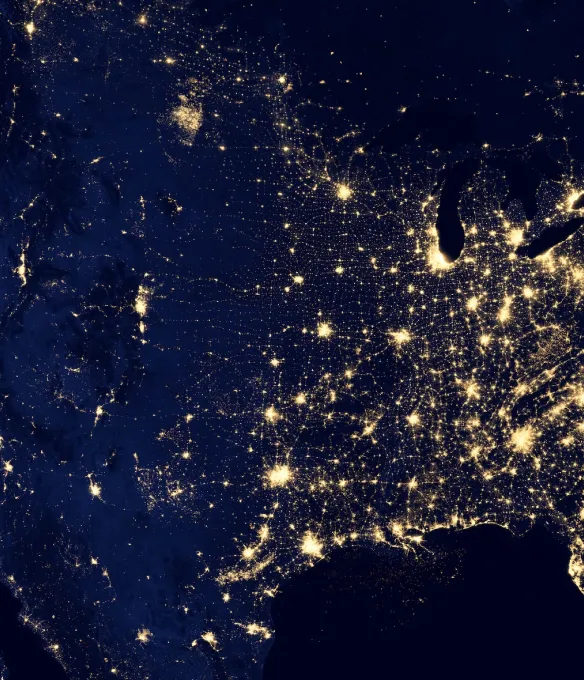
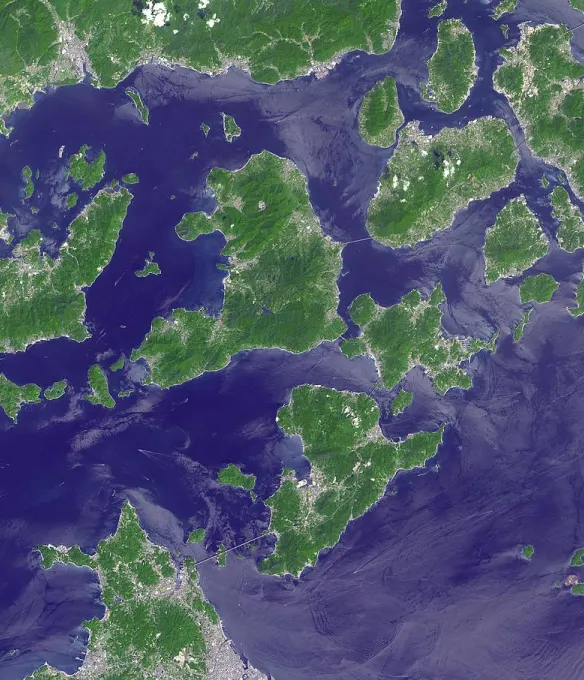

Expertise

Projects
I am part of several projects where I utilize machine learning and remote sensing across different ecosystems and application areas, including mountainous watersheds, agriculture, and terrestrial-aquatic interface.
Watershed Function
While watersheds are recognized as Earth's key functional unit for assessing and managing water resources, predicting their behavior remains a significant challenge.
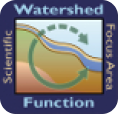
AR1K - Smart farm research consortium
Our challenge: Improve the livelihood of farmers by optimizing soil amendments with biology and data science and managing the crop cycle to enhance soil fertility.


Latest news

New project awarded
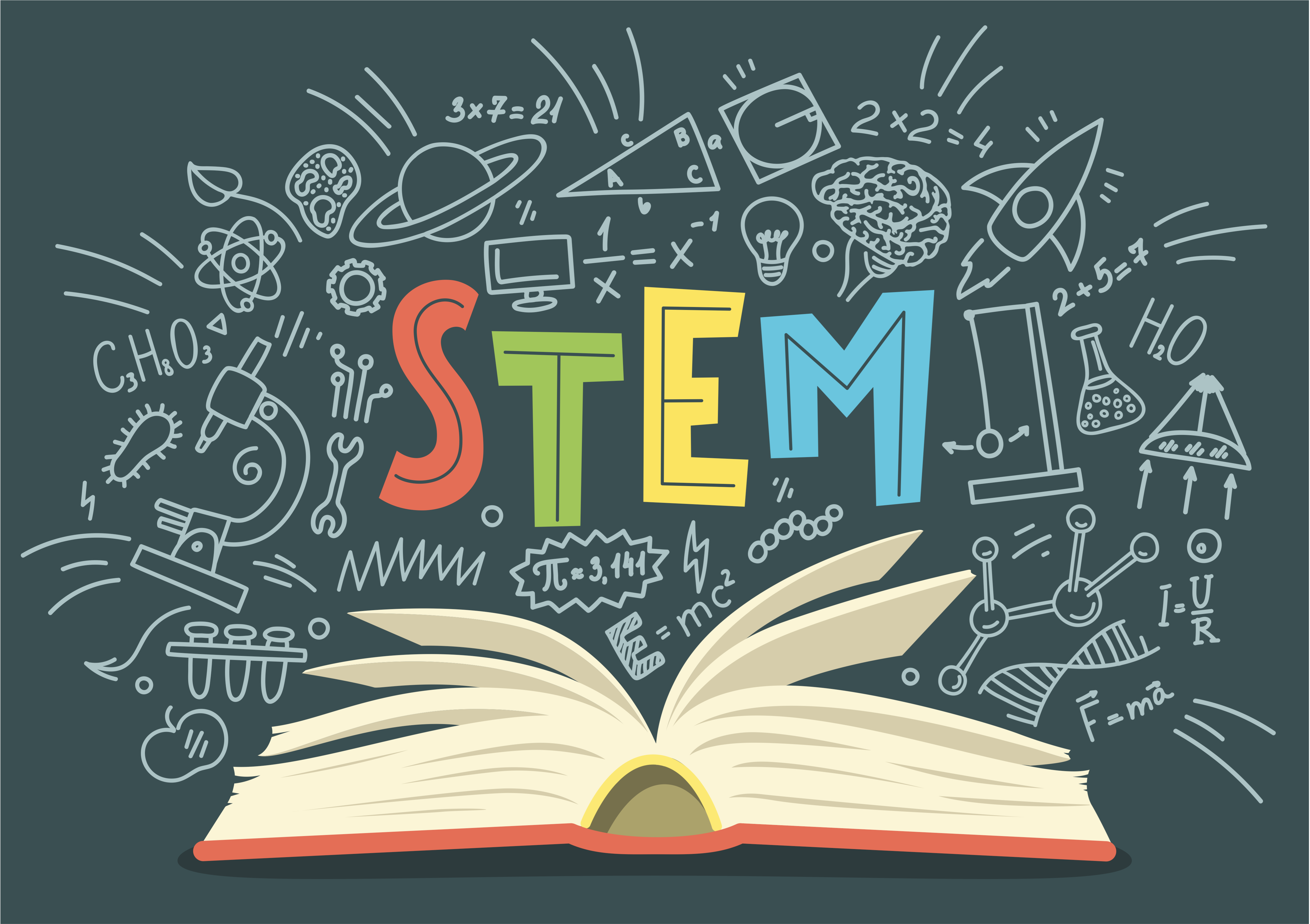
SULI program Summer '23
Publications
Diverging Climate Response of Corn Yield and Carbon Use Efficiency across the U.S.
In this paper, we describe constructing an algorithm and providing an open-source package to analyze the overall trend and responses of both carbon use efficiency (CUE) and corn yield to climate factors at the continental scale. Our algorithm enables automatic retrieval of remote sensing data through the Google Earth Engine and USDA agricultural production data at the county level across the United States through Application Programming Interface (API). We (1) integrated satellite images of MODIS-based net primary productivity (NPP) and gross primary productivity (GPP), and ECMWF-based climatic variables, (2) calculated CUE and commonly used climate metrics, and then (3) investigated the spatial heterogeneity of the variables. We applied a random forest algorithm to identify the key climate drivers of CUE and crop yield, and estimated the responses of CUE and yield to climate variability using time series data in each county across the United States. Our results show that growing degree days (GDD) has the highest predictive power for both CUE and yield, while extreme degree days (EDD) is the least important explanatory variable. We also found that yield increases with greater GDD and precipitation in most areas of the United States, but with more mixed and fragmented interactions in the southern regions—and that CUE decreases with GDD in the northern regions but increases with GDD in the southern ones. As global warming increases, both carbon sequestration and yield will increase in the south, though CUE will decrease in the north.
Advanced Monitoring of Soil-Vegetation Co-Dynamics Reveals the Successive Controls of Snowmelt on Soil Moisture and on Plant Seasonal Dynamics in a Mountainous Watershed
Evaluating the interactions between above- and below-ground processes is important to understand and quantify how ecosystems respond differently to atmospheric forcings and/or perturbations and how this depends on their intrinsic characteristics and heterogeneity. Improving such understanding is particularly needed in snow-impacted mountainous systems where the complexity in water and carbon storage and release arises from strong heterogeneity in meteorological forcing and terrain, vegetation and soil characteristics. This study investigates spatial and temporal interactions between terrain, soil moisture, and plant seasonal dynamics at the intra- and inter-annual scale along a 160 m long mountainous, non-forested hillslope-to-floodplain system in the upper East River Watershed in the upper Colorado River Basin. To this end, repeated UAV-based multi-spectral aerial imaging, ground-based soil electrical resistivity imaging, and soil moisture sensors were used to quantify the interactions between above and below-ground compartments. Results reveal significant soil-plant co-dynamics. The spatial variation and dynamics of soil water content and electrical conductivity, driven by topographic and soil intrinsic characteristics, correspond to distinct plant types, with highest plant productivity in convergent areas. Plant productivity in heavy snow years benefited from more water infiltration as well as a shallow groundwater table depth. In comparison, low snowpack years with an early first bare-ground date, which are linked to an early increase in plant greenness, imply a short period of saturated conditions that leads to lower average and maximum greenness values during the growing season. Overall, these results emphasize the strong impact of snowpack dynamics, and terrain and subsurface characteristics on the heterogeneity in plant type and seasonal dynamics.
Assessing Probability of Failure of Urban Landslides through Rapid Characterization of Soil Properties and Vegetation Distribution
Landslides are a major natural hazard, threatening communities and infrastructure worldwide. The mitigation of these hazards relies on the understanding of their causes and triggering processes, which depends directly on soil properties, land use, and their changes over time. In this study, we propose a novel framework to estimate the probability of failure in highly developed urban areas. The framework combines remote sensing and geophysical data to estimate soil properties and land covers. Such estimate properties are then integrated into a hydrogeomechanical model to provide a robust estimate of the probability of failure. To assess the importance and sensitivity of the input parameters to the probability of failure assessment, a sensitivity analysis was performed on the seven main parameters (density, friction angle, cohesion, soil thickness, slope, water recharge and saturated hydraulic conductivity) of the hydro-geomechanical model. Slope angle, soil thickness and cohesion are shown to be the most important parameters. While the slope angle can be derived from high-resolution digital elevation models, soil thickness and cohesion cannot be assessed. To incorporate the variability of these two parameters into the model, seismic noise measurements were performed to estimate soil thickness. Supervised classification of remote sensing data was used to map vegetation type and related root cohesion, which can impact the cohesion significantly. The results show that slopes with relatively thick soil layers (above 2 m) have up to four times higher probability of failure. Slopes with tall vegetation cover, and hence comparably high root cohesion, reduce the probability of failure, particularly when the soil layer is relatively thin (< 3 m). The developed approach makes use of rapid to acquire geophysical and easily to obtain remote sensing data, and hence is transferable to other study sites. This approach may be of particular importance to areas of active vegetation management that may cause considerable changes in landslide hazard maps.